This is a guest opinion piece written by Guiseppe Maio, and Jedrzej Czarnota PhD. Their biographies can be found below this post.
Open Knowledge International’s report on the state of open data identifies the main problems affecting open government data initiatives. These are: the very low discoverability of open data sources, which were rightfully defined as being “hard or impossible to find”; the lack of interoperability of open data sources, which are often very difficult to be utilised; and the lack of a standardised open license, representing a legal obstacle to data sharing. These problems harm the very essence of the open data movement, which advocates data easy to find, free to access and to be reutilised.
In this post, we will argue that data aggregators are a potential solution to the problems mentioned above. Data aggregators are online platforms which store data of various nature at once central location to be utilised for different purposes. We will argue that data aggregators are, to date, one of the most powerful and useful tools to handle open data and resolve the issues affecting it.
We will provide the evidence in favour of this argument by observing how FAIR principles, namely Findability, Accessibility, Interoperability and Reusability, are put into practice by four different data aggregators engineered in Indonesia, Czech Republic, the US and the EU. FAIR principles are commonly utilised as a benchmark to assess the quality of open data initiatives and good FAIR practices are promoted by policymakers.
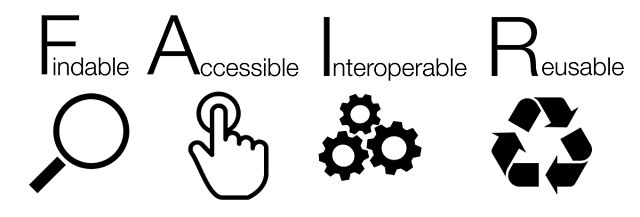
We will also assess the quality of aggregators’ data provision tools. Aggregators’ good overall performance on the FAIR indicators and the good quality of their data provision tools will prove their importance. In this post, we will firstly provide a definition of data aggregators presenting the four data aggregators previously mentioned. Subsequently, we will discuss the performance of the aggregators on the FAIR indicators and the quality of their data provision.
Data aggregators
Data aggregators perform two main functions: data aggregation and integration. Aggregation consists of creating hubs were multiple data sources can be accessed for various purposes. Integration makes reference to linked data, namely data to which a semantic label (a name describing a variable) is attached in order to allow for the integration and amalgamation of different data sources (Mazzetti et al 2015, Hosen and Alfina 2016, Qanbari et al 2015, Knap et al 2012).
Following on that, two strengths characterise data aggregators. Firstly, aggregators implement the so-called “separations of concern”: this means that each actor is responsible for a functionality. Separation of concerns spurs accountability and improves data services. Secondly, aggregators host added-value services, i.e. semantics, data transformation, data visuals (Mazzetti et al 2015). However, aggregators face a major challenge as they represent a “single point of failure”: when aggregators break down, the whole system (including data providers and users) is put in jeopardy.
In this post we investigate the Indonesian Active Hiring website, the Czech ODCleanStore, the US-based Data.gov and the EU-funded ENERGIC-OD.
- The Active Hiring website is a portal that monitors job hiring trends by sector, geographical area and job type. The platform utilises open and linked data (Hosen and Alfina 2016).
- ODCleanStore is a project that enables automated data aggregation, simplifying previous aggregation processes; the website provides provenance metadata (metadata showing the origin of the data) and information on data trustworthiness (Knap et al 2012).
- Data.gov is a platform that catalogues raw data, providing open APIs to government data. This portal is part of the Gov 2.0 movement.
- ENERGIC-OD (European Network for Redistributing Geospatial Information to user Community – Open Data) is a European Commission-funded project which aims to facilitate access to the Geographic Information System (GIS) open data. The project built a pan-European Virtual Hub (pEVH), a new technology brokering together diverse GIS open data sources.
FAIR indicators and quality of data provision to evaluate data aggregators
FAIR principles and the quality of data provision are the criteria for the assessment of open data aggregators.
Findability. Data aggregators by default increase the discoverability of open data, as they assemble data in just one site, rendering it more discoverable. Aggregators, however, do not fully resolve the problem of lack of discoverability: they merely change the nature of it. While before, findability was associated with technical problems (data was available but technical skills were needed to extract it from various original locations), now it is intertwined to marketing ones (data is in one place, but it may be that no one is aware of it). Aggregators thus address the findability issues but do not fully resolve them.
Accessibility. Aggregators perform well on the Accessibility indicator. As an example, ENERGIC-OD makes data very accessible through the use of a single API. Data.gov ‘s proposed new unit, Data Compute Unit (DCU), provide APIs to render data accessible and usable. ODCleanStore converts data in RDF format which makes it more accessible. Finally, Active Hiring website will provide data as CSV through API(s). Aggregators show improved data accessibility.
Interoperability. All platforms produce metadata (ENERGIC-OD, Data.gov) and linked data (Active Hiring Website and ODCleanStore) which make data interoperable, allowing it to be integrated, thus contributing to the resolution of the non-interoperability issue.
Reusability. ENERGIC-OD’s freemium model promotes reusability. Data.gov data can be easily downloaded and reutilised as well. ODCleanStore guarantees re-use, as data is licensed with Apache 2.0, while Active Hiring allows visualisation only. Thus, three out of four aggregators enhance the reusability of the data, showing a good performance on the reusability indicator.
Quality of data provision. Web Crawler is used in ENERGIC-OD and Active Hiring websites. This is a programme which sifts the web searching for data in an automated and methodical way. ODCleanStore acquires data in the following ways: A) through a “data acquisition module” which collects government data from a great deal of different sources in various formats and converts it into RDF (Knap et al 2012); 2) through the usage of a web service for publishers; 3) or data can be sent directly as RDF. In the case of Data.gov, the government sends data directly to the portal. Three out of four aggregators show automated or semi-automated ways of acquiring data, rendering this process smoother.
Conclusion
This post analysed the performance of four data aggregators on the FAIR principles. The overall good performance of the aggregators demonstrates how they render the process of data provision smoother and more automated, improving open data practices. We believe, that aggregators are among the most useful and powerful tools available today to handle open data.
References
- Hosen, A. and Alfina, I. (2016). Aggregation of Open Data Information using Linked Data: Case Study Education and Job Vacancy Data in Jakarta. IEEE, pp.579-584.
- Knap, T., Michelfeit, J. and Necasky, M. (2012). Linked Open Data Aggregation: Conflict Resolution and Aggregate Quality. IEEE 36th International Conference on Computer Software and Applications Workshops, pp.106-111.
- Mazzetti, P., Latre, M., Bauer, M., Brumana, R., Brauman, S. and Nativi, S. (2015). ENERGIC-OD Virtual Hubs: a brokered architecture for facilitating Open Data sharing and use. IEEE eChallenges e-2015 Conference Proceedings, pp.1-11.
- Qanbari, S., Rekabsaz, N. and Dustdar, S. (2017). Open Government Data as a Service (GoDaaS): Big Data Platform for Mobile App Developers. IEEE 3rd International Conference on Future Internet of Things and Cloud, pp.398-403.
Giuseppe Maio is a research assistant working on innovation at Trilateral Research. You can contact him at giuseppe.maio@trilateralresearch.com. His twitter handle is @pepmaio. Jedrzej Czarnota is a Research Analyst at Trilateral Research. He specialises in innovation management and technology development. You can contact Jedrzej at Jedrzej.czarnota@trilateralresearch.com and his Twitter is @jedczar.
Giuseppe Maio is a research assistant working on innovation at Trilateral Research. You can contact him at giuseppe.maio@trilateralresearch.com. His twitter handle is @pepmaio.