Report from International Data Week: Research needs to be reproducible, data needs to be reusable and Data Packages are here to help.
International Data Week has come and gone. The theme this year was ‘From Big Data to Open Data: Mobilising the Data Revolution’. Weeks later, I am still digesting all the conversations and presentations (not to mention, bagels) I consumed over its course. For a non-researcher like me, it proved to be one of the most enjoyable conferences I’ve attended with an exciting diversity of ideas on display. In this post, I will reflect on our motivations for attending, what we did, what we saw, and what we took back home.
Three conferences on research data
International Data Week (11-17 September) took place in Denver, Colorado and consisted of three co-located events: SciDataCon, International Data Forum, and the Research Data Alliance (RDA) 8th Plenary. Our main motivation for attending these events was to talk directly with researchers about Frictionless Data, our project oriented around tooling for working with Data “Packages”, an open specification for bundling related data together using a standardized JSON-based description format.
The concepts behind Frictionless Data were developed through efforts at improving workflows for publishing open government data via CKAN. Thanks to a generous grant from the Sloan Foundation, we now have the ability to take what we’ve learned in civic tech and pilot this approach within various research communities. International Data Week provided one the best chances we’ve had so far to meet researchers attempting to answer today’s most significant challenges in managing research data. It was time well spent: over the week I absorbed interesting user stories, heard clearly defined needs, and made connections which will help drive the work we do in the months to come.
What are the barriers to sharing research data?
While our aim is to reshape how researchers share data through better tooling and specifications, we first needed to understand what non-technical factors might impede that sharing. On Monday, I had the honor to chair the second half of a session co-organized by Peter Fitch, Massimo Craglia, and Simon Cox entitled Getting the incentives right: removing social, institutional and economic barriers to data sharing. During this second part, Wouter Haak, Heidi Laine, Fiona Murphy, and Jens Klump brought their own experiences to bear on the subject of what gets in the way of data sharing in research.
Mr. Klump considered various models that could explain why and under what circumstances researchers might be keen to share their data—including research being a “gift culture” where materials like data are “precious gifts” to be paid back in kind—while Ms. Laine presented a case study directly addressing a key disincentive for sharing data: fears of being “scooped” by rival researchers. One common theme that emerged across talks was the idea that making it easier to credit researchers for their data via an enabling environment for data citation might a be a key factor in increasing data sharing. An emerging infrastructure for citing datasets via DOIs (Digital Object Identifiers) might be part of this. More on this later.
“…making it easier to credit researchers for their data via an enabling environment for data citation might a be a key factor in increasing data sharing”
What are the existing standards for research data?
For the rest of the week, I dove into the data details as I presented at sessions on topics like “semantic enrichment, metadata and data packaging”, “Data Type Registries”, and the “Research data needs of the Photon and Neutron Science community”. These sessions proved invaluable as they put me in direct contact with actual researchers where I learned about the existence (or in some cases, non-existence) of community standards for working with data as well as some of the persistent challenges. For example, the Photon and Neutron Science community has a well established standard in NeXus for storing data, however some researchers highlighted an unmet need for a lightweight solution for packaging CSVs in a standard way.
Other researchers pointed out the frustrating inability of common statistical software packages like SPSS to export data into a high quality (e.g. with all relevant metadata) non-proprietary format as encouraged by most data management plans. And, of course, a common complaint throughout was the amount of valuable research data locked away in Excel spreadsheets with no easy way to package and publish them. These are key areas we are addressing now and in the coming months with Data Packages.
Themes and take-home messages
The motivating factor behind much of the infrastructure and standardization work presented was the growing awareness of the need to make scientific research more reproducible, with the implicit requirement that research data itself be more reusable. Fields as diverse as psychology and archaeology have been experiencing a so-called “crisis” of reproducibility. For a variety of reasons, researchers are failing to reproduce findings from their own or others’ experiments. In an effort to resolve this, concepts like persistent identifiers, controlled vocabularies, and automation played a large role in much of the current conversation I heard.
…the growing awareness of the need to make scientific research more reproducible, with the implicit requirement that research data itself be more reusable”
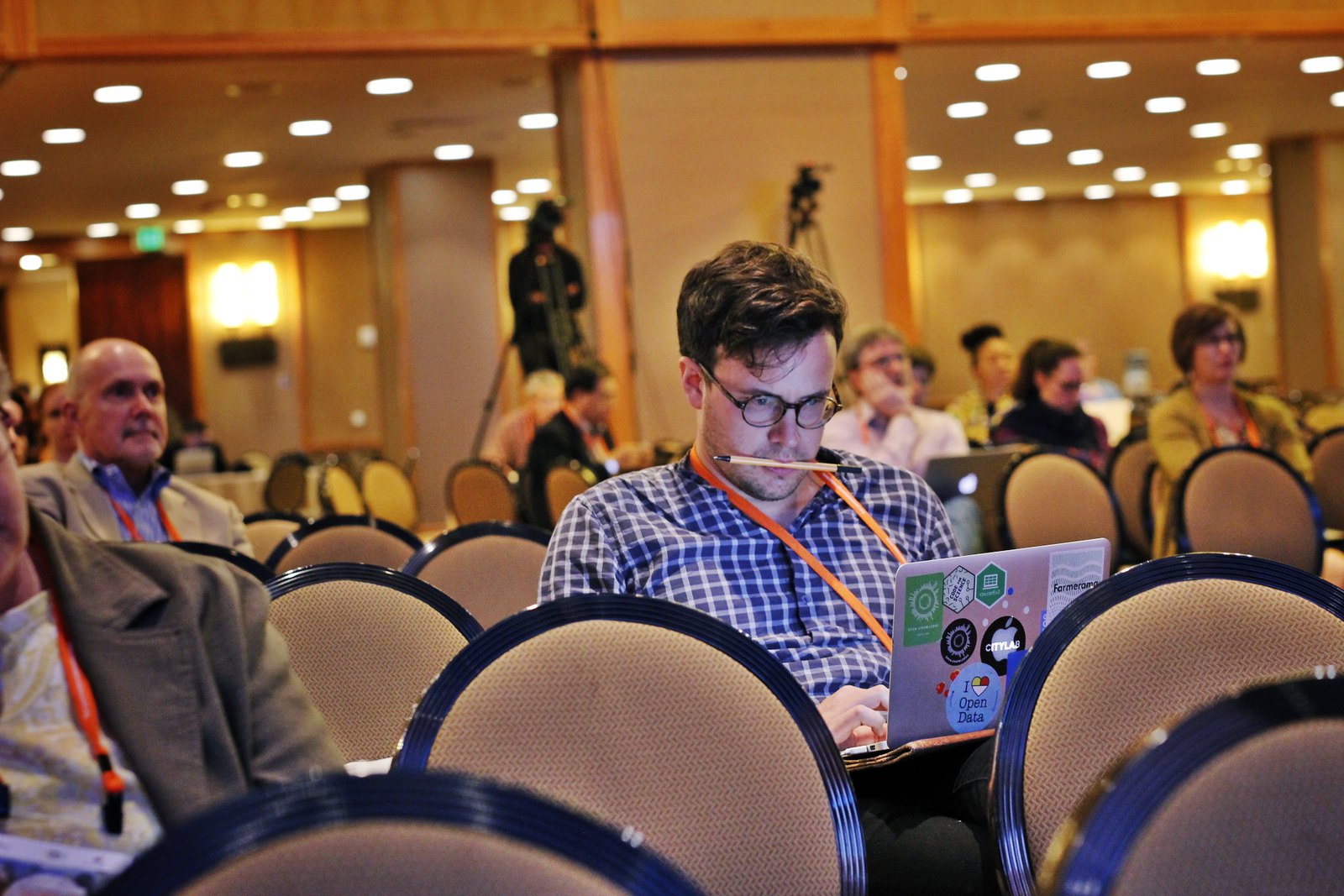
Persistent Identifiers
Broadly speaking, persistent identifiers (PIDs) are an approach to creating a reference to a digital “object” that (a) stays valid over long periods of time and (b) is “actionable”, that is, machine-readable. DOIs, mentioned above and introduced in 2000, are a familiar approach to persistently identifying and citing research articles, but there is increasing interest in applying this approach at all levels of the research process from researchers themselves (through ORCID) to research artifacts and protocols, to (relevant to our interests) datasets.
We are aware of the need to address this use case and, in coordination with our new Frictionless Data specs working group, we are working on an approach to identifiers on Data Packages.
Controlled Vocabularies
Throughout the conference, there was an emphasis on ensuring that records in published data incorporate some idea of semantic meaning, that is, making sure that two datasets that use the same term or measurement actually refer to the same thing by enforcing the use of a shared vocabulary. Medical Subject Headings (MeSH) from the United States National Library of Medicine is a good example of a standard vocabulary that many datasets use to consistently describe biomedical information.
While Data Packages currently do not support specifying this type of semantic information in a dataset, the specification is not incompatible with this approach. As an intentionally lightweight publishing format, our aim is to keep the core of the specification as simple as possible while allowing for specialized profiles that could support semantics.
Automation
There was a lot of talk about increasing automation around data publishing workflows. For instance, there are efforts to create “actionable” Data Management Plans that help researchers walk through describing, publishing and archiving their data.
A core aim of the Frictionless Data tooling is to automate as many elements of the data management process as possible. We are looking to develop simple tools and documentation for preparing datasets and defining schemas for different types of data so that the data can, for instance, be automatically validated according to defined schemas.
Making Connections
Of course, one of the major benefits of attending any conference was the chance to meet and interact with other research projects. For instance, we had really great conversations with Mackenzie DataStream project, a really amazing project for sharing and exploring water data in the Mackenzie River Basin in Canada. The technology behind this project already uses the Data Packages specifications, so look for a case study on the work done here on the Frictionless Data site soon.
There is never enough time in one conference to meet all the interesting people and explore all the potential opportunities for collaboration. If you are interested in learning more about our Frictionless Data project or would like to get involved, check out the links below. We’re always looking for new opportunities to pilot our approach. Together, hopefully, we can make reduce the friction in managing research data.
- Newsletter: http://frictionlessdata.io/get-involved/#newsletter
- Specifications: http://specs.frictionlessdata.io/
- User Stories: http://frictionlessdata.io/user-stories/
Dan Fowler contributes to various projects at Open Knowledge and currently serves as developer advocate helping to connect a community of makers and doers around open data with the technology work conducted by Open Knowledge International. He has a Master’s degree in Information and Communication Technologies for Development from Royal Holloway, University of London and a Bachelor’s degree in Psychology from Princeton University. Between degrees, he worked as a sysadmin for an investment bank in New York.